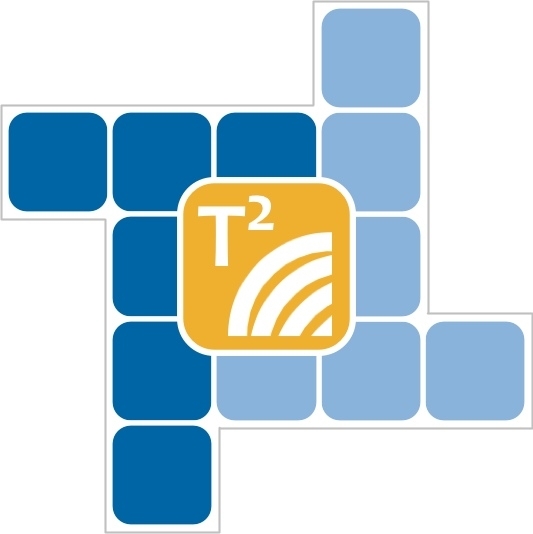
T-Squared: a Teaching and Technology Podcast
Season 3, Episode 3: A Dose of Technoskepticism with Eric Covey
Join the conversation!
This episode transcript has been enhanced with social annotation tools from Hypothesis. By creating a free account you can highlight text in this transcript and add your own comments or questions. Other users will be able to see your annotations and respond in kind.
For more information, please visit they Hypothesis Quick Start guide.
Matthew Roberts
So. Hi, Jacob.
Jacob Fortman
Hey. Nice to be here.
Matthew Roberts
Yeah. So this is like déja vu, because when we had our recent conversation with Eric Covey, doctor of—you don't say doctor of history. Do you say Professor of history—Dr. Eric Covey. We had such a great conversation about issues related to ethics, which spanned so much territory. We wanted to make sure that we had another episode. So we are back. The conversation continues. So I'd like to welcome back Eric Covey to to our podcast today. And let's pick up things where we left them last time.
Siri
On the last episode of T Squared. The conversation had turned to the ethical challenges facing generative artificial intelligence. In particular. Can AI's electrical and cooling needs find a solution through technology itself? And until those problems can be minimized, how do we handle the fiscal and environmental costs they impose? Let's pick things up with Eric Covey talking about the similar environmental problems arising from cryptocurrency.
Eric Covey
You know, if we're thinking about crypto as the previous or one of the kind of previous environmental technological disasters, you know, Etherium I think, changed how they verified Bitcoin transactions and saved like 90% electricity. I don't I don't know if Bitcoin's done that at this point, but Bitcoin is, you know, I guess not as consuming tremendous amounts of electricity. And the question too becomes, you know, who—you know—there are environmental consequences, you know, whether they're solved in the long term or not, you know, where are those environmental consequences most likely to take place?
Right? They're obviously most likely to take place in places with weak regulatory enforcement mechanisms for environmental regulations.
But, you know, there needs to be a kind of like supranational thought given to this. You know, one of the examples given in one of these articles, right, is that it's much cheaper for a data center to be cooled in like Microsoft's Washington data centers, for instance. Right. Or much, much cheaper and more kind of environmentally responsible to cool those than if Microsoft builds data centers in Southeast Asia, for instance, which might offer tremendous kind of financial incentives and in fact, might even benefit the economies, you know, you know, Southeast Asian nations.
But the environmental consequences are like five times worse. It takes like, you know, all of this kind of additional electricity and water to cool these facilities. So, I mean, this comes back to the question of kind of the environmental crisis in general. Is this something that can be solved? I don't know. It definitely can't be solved by universities. Could it be solved by individuals? No, it can't even be solved by nation states. So, like, what is the what's like the larger movement it takes to, you know, incorporate technology in an environmentally and kind of, you know, human ethics responsible way?
Matthew Roberts
My background is as a political scientist. So, I mean, instinctively I started thinking about, well, you know, public opinion is one thing, but what is the— what is the lever or the mechanism that turns that into action? How is it that I mean, we then have the momentum, have the impetus to not just be frustrated by the situation or to march in the streets about it, but I mean, how do we actually then translate that into action actually happening?
Eric Covey
Have we asked have we asked what the answer to that question is?
Matthew Roberts
Okay. So because we need a gimmick. No, we don't need a gimmick, but because why not? I'm not going to give it a microphone. But we will do that here. I think you were being flippant.
Eric Covey
Now, I was maybe more serious than I was being flippant.
Matthew Roberts
Okay. So I have not paid them for ChatGPT 4
Eric Covey
Contra some of my students, who regret spending the money.
Matthew Roberts
Do they?
Eric Covey
I'll tell you about that if you'd like. Okay. I have.
Matthew Roberts
I have just not pulled the trigger because to be honest, the price doesn't seem worth it.
Eric Covey
To me either either.
Matthew Roberts
So I'm stuck with ChatGPT 3.5.
Eric Covey
A dinosaur.
Matthew Roberts
Yeah. So it's answers might not be as good.
Now. Maybe I should be pulling up Bard or something because I have trying more of them.
But let's try here. So what question do we want to ask?
How do— how do we—how do we make people—how do we create change in environmental policy
Eric Covey
At a at a supranational level?
Matthew Roberts
How? Please give me see, I have not taken the places on prompt engineering, you know, please give me five ideas for how to. I hope my typing sound picks up on the microphone because that always makes it more impressive, right? You can tell I'm actually doing this. Please give me five ideas for how to create international policy changes in regard to the climate crisis.
Now, to change that, gentlemen, because this answer here is going to determine how we solve our—we need to get the prompt right.
Eric Covey
Read it back again.
Matthew Roberts
Please give me five ideas for how to create international policy changes—to create international policy changes in regard to the climate crisis. I'm not happy with that, but I'm never happy with what I wrote.
Eric Covey
I think that's good enough. We don't want to get too too much back. And I also wonder how many of us use please when we query the LLM.
Matthew Roberts
My wife is a strong believer in always asking our Amazon Echo with please. And I do have an Echo device here on my desk, so I'm not going to say the magic word because I think we did have that in the last episode, right? I think she responded when I said Alexa probably at some point.
Eric Covey
And we should—we could get into the kind of gendered aspects of this responsive technology, too, Right? Why are we so often interacting with a woman's voice? Why is—why is—why is you know, why are these like pink collar? Why is the AI doing so much pink collar work.
Jacob Fortman
Siri and Alexa...
Matthew Roberts
My wife is very very fond of having changed Siri on her Apple Watch to be an Australian male.
Eric Covey
Oh, interesting. Okay.
Matthew Roberts
Oh, this is this is interesting. So here is the response. Creating international policy changes to address the climate crisis requires a multifaceted approach that involves cooperation among nations. Here are five ideas. Oh, I thought it was hung because it actually paused there for a good 15 seconds. So apparently it had to think really hard.
Eric Covey
You just you just consumed 500 milliliters of water with that query.
Matthew Roberts
I think that was a 750 milliliter.
Eric Covey
Wow.
Matthew Roberts
But let's see, I'm not going to read this whole thing because hopefully, dear listener, you know, we're a little tongue in cheek here, but. Global carbon pricing mechanism, international climate agreement trengthening. The way you get the agreement is by getting the agreement, right. And that's the essence. Technology transfer and capacity building. Conservation and reforestation programs, climate resilience funding.
Eric Covey
So. So perhaps we could blame, you know, our lack of kind of proper prompt engineering. But, you know, ChatGPT seems to know nothing about politics or power. Well, in its response.
Matthew Roberts
I'm convinced that one of the most important things we need to do in the academy when it comes to generative artificial intelligence is promote the digital literacy aspect of this. We can't make everybody into Python programmers and we don't need to. But the fact is, the reason why ChatGPT doesn't know about politics is because—and I realize there are computer scientists who claim they will know more about this than than we do. And I'm willing to give them their area of expertise because I do believe in the concept of academic expertise. But knowledge is not an emergent property of hundred billion parameter large language models. It does not actually know something.
And I mean, one of the best examples and maybe we're transcending the topic for today. I mean, one of the things that has impressed me by which I mean not make me wow, that's cool, but has been pressed onto me. The original sense of impressing—about large language models is that they're a form of compression. The concept—and sorry to go all technical here. If this is not your thing. But, you know, back in the day when we had dial up and the fact is we didn't have huge storage, right? The pictures we had online sometimes were bad looking, and that's because we needed to use software that would reduce the size of these images. Now we send around the six megabyte snaps from our phones, which is no big deal, and they transfer really quickly.
Back in the day, oh I'm sounding old, back in the day, we couldn't do that, right? We still have compression it's important in things like movies and all that, right? You still don't want your 4K video file to actually take 50 gigabytes, right? Because it'll still blow through your data cap on your home Internet. But that's what large language models themselves are like. And this is true for large language models, but also for image generating AIs.
The fact is they consume all that input information and their goal is to reduce it to a smaller size that is as faithful as it can be to whatever the nuance and complexity is that's in that original data. If the content of the large language model was as large as all the data it pulled in, it would not be revolutionary. It would not be that interesting. But the idea is that if you take it all in, you boil it down on the stove of computer science, and you do away with some of the excess that's not really in there, right? I mean, that's you know, there are areas in the photo of your camping trip— you don't need 15,000 shades of blue in that one area of the lake when 5000 shades of blue will do. Right? If you can get rid of some of that detail, then you've saved space.
That's how generative AI operates. But the fact is, not only does it not have knowledge in a sense, but it doesn't truly have creativity. And, we can come back to this in a future episode and we will. And maybe in two years I'll be proved wrong. But the reason why this does not read like an innovative list of policy proposals is because it's bound by what it sucked into its data. So if no human being came up with the brilliant solution and that brilliant solution was sucked into the training data, it's not going to get spat out. And let's—and let's, you know, call spades spades. Right. You can get you can get generative AI to do impressive acts of pseudo creativity.
I was joking around with Eric before you got here, Jacob. About recipes, right? I mean, you can ask ChatGPT to give you a recipe. The thing is, just coming up with a recipe doesn't mean it's creative. I mean, it's largely just a reassembling language components that it's found in other recipes. I mean, hopefully people will still someday want to go to a restaurant of a well-known chef because they have come up with something that is truly inspired—that is a combination that no one would think of, that didn't arise because you took predictable things. Like if you're making a chili recipe, it's not creative if you say one ingredient should be ground beef and another should be tomatoes. And but, you know, even throwing in a random element.
I mean, for those of us who do dabble in programing for a long time, when you wanted things to seem creative, you just made them random. Right. So you have a long list of things—you have a list of 15,000 ingredients and you throw one randomly in. Right? So my recipe for chili is ground beef, tomatoes, chili peppers, and kumquats. Okay. Well, you know, random kumquats. Now, I do have to defer here because Eric has some experience in the area of Texas barbecue, which I—did I say I was not going to bring it up? But I mean, maybe you have a secret recipe involving kumquats that, you know, is really good.
Eric Covey
You didn't you didn't promise me that. I mean, I can imagine a way in which kumquats could be incorporated quite well in barbecue. I do have two things, though. All that stuff you just said. So, number one, you know, like, of course, it's all well and good that this large language model is able to do this incredible thing, to take all this kind of data and boil it down and give it back in a comprehensible way. But number one, we don't know what data it's boiled down, right? Like, we know, you know, web crawlers are kind of, you know, going out and kind of getting information on the Internet and then, you know, open AI— what's the Web crawler, the big one that OpenAI has used mostly?
Matthew Roberts
Oh, I forget.
Eric Covey
You know, I don't remember either at this point. So they're they're not like this isn't all of human knowledge. Right. This isn't even what's on JSTOR. This is just all this random junk on the Internet. And then, of course, like part of the model collapses that the AI increasingly becomes trained on itself, which is just kind of nonsense and kind of mediocre stuff like this.
There's inherent problems with the data set itself that it's boiling down from. Right. And we now, you know, Kenyan workers scrubbing, you know, really offensive content from these large language models, data sets. You know, in one instance, there's this company in San Francisco, Samar, they do a lot of outsourcing. One of their outsourcing gigs was with OpenAI paying paying Kenyan workers $2 an hour to essentially go over, I think, like between 100, 250, you know, pieces of kind of data every day. One worker talks about the kind of nightmares he experienced after having repeatedly reading, you know, a description of a man having sex with a dog in front of a child. Right. So that's the kind of material they're trying to scrub this out of this these large—
Matthew Roberts
Thanks Eric. This podcast is now officially parental guidance required.
Eric Covey
What gets past those kind of of human human censors scrubbers right like so Lyon, this German company, right they have this—they use the data set, they have a program that converts text to images so it's been trained on captions, texts or images from the Internet. And they've got this publicly available data set out there. And, you know, not too long ago, what was discovered is that among the 5 billion images in this dataset were a thousand images of child sexual abuse. What these kind of large language models, these kind of image generating programs are being trained on is not just like Internet garbage, but also some kind of like really offensive content sneaking through.
And I have one other thing to say about this. I don't remember what it was. You said so much.
Matthew Roberts
I'm sorry.
Eric Covey
Kumquats, and uh, the recipe? Goodness.
Matthew Roberts
You think about it, I'll make a I'll make a point before I forget my point. Oh, it's already slipping. Your comment about the training data there is a really good one because it points out what a lot of people haven't necessarily thought about, which is there are two faces of bias in training data for generative AI, and these are the biases that limit what can come out of it.
So the first is the obvious bias that's present in the data, right? So to the extent that there is offensive content, whether it's offensive in terms of sexuality and violence, whether it's offensive in terms of white nationalism, right. I mean, please stop training your language models on 4Chan. But to the extent that there is that kind of bias in the training data, it is going to be overrepresented in the output, which is to say that output will be biased because of the inputs contains stuff, that stuff will come out. That's the kind of active bias.
And then the other kind of bias is also important to us, I think, in the educational sphere, and that is kind of a passive bias or an absence bias. I'm thinking on the spot here. I don't have terms for these, but and this is where I think we as educators should be uniquely concerned because this is the less obvious kind of bias.
So one of the things that I think is a hallmark of us as educators is frequently the things that we consider important or the things that we want to bring students' attention to are not the things that are obvious, right? You know, you can go to wherever and find, you know, who the hundred greatest authors in American literature art, right? I think if you're a good American literature professor, what you put on your syllabus is not "here's the 100 greatest"—you put the "nobody knows about this person, but here's why they're important."" Here's why there is a value to studying them, right?
As educators, we most frequently focus upon the small, the marginalized, I mean, marginalized in every sense. And those are the things that we bring to the attention of those that we help educate. To the extent that those things are already minority positions, right, in a mathematical sense, to the extent that they are minority positions, they will be underrepresented in training data. And then frequently, because the process of using training data is a process of compression, which means and to be clear, this is what technical people called lossful or lossy compression. Information is lost in the process of compressing all this knowledge. Those minority viewpoints will, I believe, I think I'm justified in believing it—will be more likely to be not just underrepresented, but literally disappear from the training data as a result of the compression.
Eric Covey
What does ChatGPT not know. Right, as a result of that compression. What is lost?
Matthew Roberts
Yeah, going with cultural connotations. I'm in the middle of watching the second season of the Foundation TV series that Apple TV is putting out based upon Isaac Asimov's Foundation and all sorts of books. Anybody else? Hmm. No? Okay, I'm enjoying it. I love the books and there's a lot of differences from the books, so I'm trying to, you know, keep it clear. But one of the things that's coming up time and time again is the issue of memory and knowledge enforced forgetting. And what does it mean to have things taken away from you in terms of your knowledge, your memories, and to not even know what happened? Right. I don't want ChadGPT, Bard, Claude or anybody else writing a syllabus for me because the things it's going to generate do not include the things that I probably think are most important. There will be syllabus differences from my class and the similar class taught at AIU.
Eric Covey
You know, and it's interesting too, because for so long, you know a lot of kind of—for so long a lot of kind of the academy and professors' criticisms of technology online stuff—Wikipedia is the common one right. Don't use Wikipedia. That's a whole other conversation. But I tell students, you would be so much better off learning about a topic from Wikipedia than you would from ChatGPT. You know, it's such and that's a kind of interesting moment, too, I think, in and of itself, right, that suddenly Wikipedia is a much better source than this kind of like new technology that's being heralded as revolutionary.
Matthew Roberts
Going back to the the digital literacy thing that I had brought up a few moments ago. I think that's an excellent point, because Wikipedia is the great ironic example. When it burst onto the scene as academics, I think we were grateful to be skeptical about this concept of a crowdsourced encyclopedia because most of us have met the crowd. We are the crowd. We know ourselves, right? But the ironic thing is, over time, Wikipedia—and there have been tests to verify this—that the content accuracy of what's in Wikipedia is amazing. And, you know, let's throw out all the same standard arguments about bias and whatever. That's true for any source of information. But the point is that Wikipedia evolved in a direction that I don't think we necessarily anticipated, which is that the people who do most of the Wikipedia, Wikipedia editing are actually informed about their topics, and they are darned interested in making sure that the content is accurate.
Eric Covey
And when you're thinking or talking a few moments ago about minority positions too, it's been especially in those kinds of spaces in universities where there are Wikimedia, you know, kind of editing sessions, right? Or what are they called—editing marathons? You know, so like in the field of Black Studies, for instance, you bring a whole bunch of kind of scholars and students and people interested in that together. And you spend time updating, creating, and editing, you know, kind of entries on famous kind of people, movements, moments, places in black history. Right? So I think to some degree it's actually been Wikipedia, and that kind of public space has been embraced now by some people in the university.
Matthew Roberts
And you're saying that in the mental image that came to me, was that I mean, compared to what we just said about large language models being a concept of compression, you're describing a process of, I have to say, decompression, right? Because the point is that the knowledge represented in Wikipedia is actually expanding and growing.
But I think the point that I had been hoping to make and this is something that I'm still trying to figure out what term I want to use for this, because I think it comes back to many of the things we've been talking about, which is this concept of good enough, which is a concept of who do we defer to for knowledge or action, Right? You could call it credence. You could call it credibility. I don't know. And you referenced my comment about ChatGPT doesn't have a CV, right? That's true. But, you know, Wikipedia, the thing that—the thing that has made it not to be the black sheep of of the academic world any longer is the fact that you can follow that, right? I mean, you can you can see who edited it. And I mean, even if they're hiding behind some some handle, which is kind of obtuse. I mean, there have been studies that show that there is a credibility to it. Nothing at the moment, because it's not a technical problem, as far as I can see, shows that that will ever change about generative artificial intelligence.
Eric Covey
The Wikipedia, Wikimedia, the Wikimedia Foundation in general has largely also avoided being kind of corporatized, right? Like it still runs on individual donations and I still get those emails and, you know, so it isn't you know, it's kind of responsive to, you know, not the kind of neoliberal imperatives of the university or of corporations. And, you know, I think it is really important, like you said, that this is—the data set;s visible. You know, you can see the kind of changes that have been made. You can see even if they're anonymous, the people who are kind of editing that and that kind of transparency, you know, just isn't there with these large language models.
And I would be—I would be kind of remiss, I feel like, too, if, you know, there's a lot of talk, I think, also about so-called kind of, not open AI like the company OpenAI, but open AI models that are kind of more available, perhaps smaller. But back in 2009, Michelle Thorne coined this phrase open washing to describe those kinds of things, like, in fact, most of these models, even if they're, you know, quote, open AI models, are still being developed by companies or for companies with their kind of needs in mind and, you know, don't really ever mean to do the same kinds of things that something like Wikimedia does or Wikipedia.
Matthew Roberts
Yeah, thanks for bringing that up, because I was thinking about pivoting to that point as well. This question of of the term open, which has a broader history in terms of the technology industry. Yeah. So this is a quote in one of the articles that you assign to your students, which gets at some of this. Right. "There's a really big difference between saying that open source is going to democratize access to AI and open source is going to democratize the industry." Sarah Meyers West, the managing director of the AID Now Institute, told me—me being the the author of this selection who is a Mateo Wong.
Yeah. I mean, there's a lot to be said there about this question of open, because in some ways the fundamental nature of generative AI is that it simply cannot be open. I mean, you can scrutinize the source code for for the front end, even for some portions of the back end, if, if the companies will let you see it. And this is you know, I want to try to tread lightly here in not being guilty of some sort of, you know, techno mysticism. But the way that these very large neural networks work to generate what they're capable of are processes that are perhaps fundamentally inscrutable to the humans who have created them.
I mean, you can find the computer scientists working in these companies saying, we don't really know how it makes these connections. The layers of neurons, the billions, hundreds of billions of parameters. So soon, trillions of parameters. We don't know what they are. We don't know how they're operating. And to be honest, it's hard to sift through a trillion data points. Right? I mean, I don't know what visualization you would be able to create that would allow you to understand what it's doing. So, I mean, for one point, it's simply impossible for generative AI to really be open.
But, I mean, there's practically—there's more practical ways that we could talk about that. I made a note here at the end of that article, because, you know, we talked about, you know, the technical challenge of the environmental impacts of technology. I mean, you could talk about other technical challenges that are involved as well that could get solved over time. But the fact is and I don't think anyone is saying differently here, if generative AI is going always to inherently be costly both to develop and run, is it reasonable to ever expect that as a technology, it can ever be fully separated from for profit entities?
I mean, Wikimedia, somehow they survive. Right. I mean, we see that banner at the top of the page asking us for money every so often. But but the fact is, as much even as the well-intentioned talk about truly open AI and to the extent that for example Meta has—what's the name of their model that they claim is open, there's still always—
Eric Covey
Llama.
Matthew Roberts
Llama. Yeah. Llama llama in the red pajamas. We could talk about you know, the the honesty in talking about being open, the idea of open washing, as you mentioned, Eric. But I mean, can we ever get away from the fact that someone has to pay for all that computing power? Even if we make it cheaper, there still will be a cost.
Eric Covey
So several things, I think. So, number one, the fact that like increasingly too, venture capital for large language models for AI is drying up and you're left with just like the major players, right. Universities can't afford to do it, you know, on any kind of really large scale. So it's like we're stuck with Meta, OpenAI, which is Microsoft, right? So there aren't any other kind of players to develop something different.
Matthew Roberts
Google and Anthropic.
Eric Covey
Oh, Google. Yeah, Google, of course. Anthropic. Yeah. But I mean, you know anybody. There's no there's nothing new on the horizon. There's like no new company going to open up. I mean, OpenAI is so interesting too, because their initial mission is like, we're going to change the world and we're going to release everything and give it to everybody for free. And then now they're kind of changing, their updating those terms of service, those terms of kind of employment. They've got a new, a new, more valuable mission. And then the inscrutability too, like no one understands how these things actually work. I mean, it seems like maybe that should be frightening.
One of the things my partner Roberta, wanted me to tell you all at the beginning of today was that John Connor would be very disappointed in all of us at this point.
Matthew Roberts
I tried not to pull any Skynet references. I am trying to be on the clear side of that Chicken Little version of things.
Eric Covey
I mean, it's hard not to I mean, the mediocrity element. I think I'm going kind of a little off script here.
Matthew Roberts
There is no script.
Eric Covey
What we're talking about. I mean, I do want to I do want to talk about that, though. So one example. I had two students last semester who, in spite of the workshop where I say if you can find useful ways in the work in the class to use these LLMs to use ChatGPT. Let me know. Just be transparent about it, because I'd be curious to find out.
So I had two students who used it and didn't tell me, of course. And when I read their work, it was apparent right away that this was from a large language model. And I invited them both to come to talk to me in my office. And without going too much into the kind of demands of the assignment, one of the things is I want you to take academic articles, scholarly articles, secondary sources, and draw material from those that respond to a question. What it doesn't ask you to do is to write a description of the article, because we already have that. It's called an abstract. The authors who are familiar with their work and what it does wrote that. It wasn't you've fed the PDF into ChatGPT and it did a kind some calculations and determined what was there.
So both students had just fed these PDFs into ChatGPT and what they had provided in their assignment was descriptions, not not what I wanted. That already exists. They could have just if they were going to do that, they could have just copied the abstract. One of the students, though, what was most interesting about his assignment and he had paid for ChatGPT 4 is that as I was reading through the saying and I was like, oh yeah, you know, this was written by ChatGPT, but probably I got about three quarters of the way to it and it switched to French, you know, and for two or three sentences it was all French, entire sentences in French. And it was weird to me. So I brought it to my office and I asked him, you know, he admitted it right away what he had done. And I said, you know, first of all, he told me that it was paying for ChatGPT, you know, the $20 a month that you're unwilling to spend. And I said, Well, did you see that? You know, part of your thing was, how did this happen?
And he said, I just didn't notice it was in there. And it's still, it's unclear the inscrutability, like, why did this happen? Why in this kind of like two paragraph description, did ChatGPT suddenly spit out two, two or three sentences in French? But the mediocrity, he didn't even read what the LLM had spit out, he just copied and pasted it. It was incredible to me.
Matthew Roberts
And now we're we're now we're bound, bordering on another long conversation we could have about about things we've seen in our time teaching. Yeah, you know, they should. I think what we need to start emphasizing when it comes to even getting text from generative AI is proofreading out loud is still important, but it goes back to the good enough, right. And goes back to this question of credibility or credence or whatever we're going to call it. I mean, the presumption that I don't need to read through it because it is good enough.
Yeah. Part of this, I think, is educating about the concept of, and I really hate the term hallucinations, because I think we need to stop ascribing. We need to stop personifying it as much, because it's a marketing— I'm not saying it was invented by those in marketing. I'm not saying that marketing is bad, but so much of the use of those terms ends up influencing the way in which we think about it, and we we shouldn't think about it as hallucinations because it's kind of like, Oh ha ha, ChatGPT, you're off your meds. Sorry, not trying to be flippant about that either. But you know, it's a problem that can be solved, right?
But it's not. It's. It's endemic. But yeah, read your stuff, people. Read your stuff.
Eric Covey
I mean, it comes back, I guess, to the techno skepticism also, you know, even if this is what I was asking you to do or this was perhaps going to be useful to you, you should still be skeptical and attentive and, you know, you know of the outputs of this thing, but also the kind of like larger social, environmental, human, economic, and so forth, context that it's—that you're living in. Right. That you're kind of interacting with this technology.
Matthew Roberts
And just because we haven't connected enough dots among buzzwords and concepts, we need to make the bigger one. I mean, I think if there is any further proof, we need that the conversation from years ago about the concept of digital natives—
Eric Covey
Oh goodness.
Matthew Roberts
Remember that, was it Marc Prensky, I think. This concept that the incoming generation of students who I think now, based upon the age of that piece, I mean, we are like a generation or two past them. The idea that students coming in were somehow these digital natives who because they were raised with technology, they're they're inherently beter—there's never been any empirical validity to that claim.
And I mean, the point that I was trying to communicate based on that was, well, you know, literacy comes in multiple levels, right? And what is true is that, you know, the students we've been seeing in higher ed for the last ten years, they have a certain increased level of what I call consumptive literacy, right? I mean, but there's a big difference between being able to consume, you know, watching YouTube videos, knowing how to install the app, to watch things on the new social media network you want to be on.
But there's differences between that and the next level up, which is productive literacy, right? Because you still, you know, try to get people to use Excel or something. Well, you know, that's a foreign language. But but then the third level of that is critical literacy. And that is, I mean, what has to be developed and that requires knowledge and insight, and in the concept— context—of generative AI, guess what the need to be critical is—is ever stronger.
So I have a sense this conversation could keep going and going and maybe we need to just make Eric the permanent third, third member of our team here or something. Jacob and I would like to thank you for joining us, Eric. I think this has been a great conversation and definitely has advanced the conversation that we want to be having about generative AI and how that's impacting us both in education— after all, this is T Squared, a teaching technology podcast. But, you know, beyond that for us as members of the university community, higher education in general, and as citizens of this globe.
Eric Covey
Yeah, I mean, you know, Thank you, Matt. I said at the end of the last episode that this conversation I feel like was a long time in coming. So I've been really pleased both these times to sit down with you and Jacob. And I think also it's important to continue these conversations. So certainly anytime you all want to invite me back to talk about anything, if I have anything left to say, in fact, you know, I'd be happy, happy to be here.
Jacob Fortman
Yeah. It's been it's been a great pleasure chatting with you and chatting with you, Matt, as well. It's always it's always a great time.
Matthew Roberts
All right, well, dear listener, we release you to whatever it is that you were doing, and until next time, we will see you. See—no, I guess we won't see you. We won't hear you either. We'll just know that you're quietly there lurking on T squared a Teaching and Technology podcast.
Siri
T-Squared. A teaching and technology podcast is produced at Grand Valley State University. Our theme music is from Bill Ryan and the Grand Valley State University new music Ensemble. The views and opinions expressed in this episode are those of the speakers and do not necessarily reflect those of any institution or artificial intelligence. This podcast does not take an official position on whether Australian Siri is in fact the coolest Siri.
T-Squared.